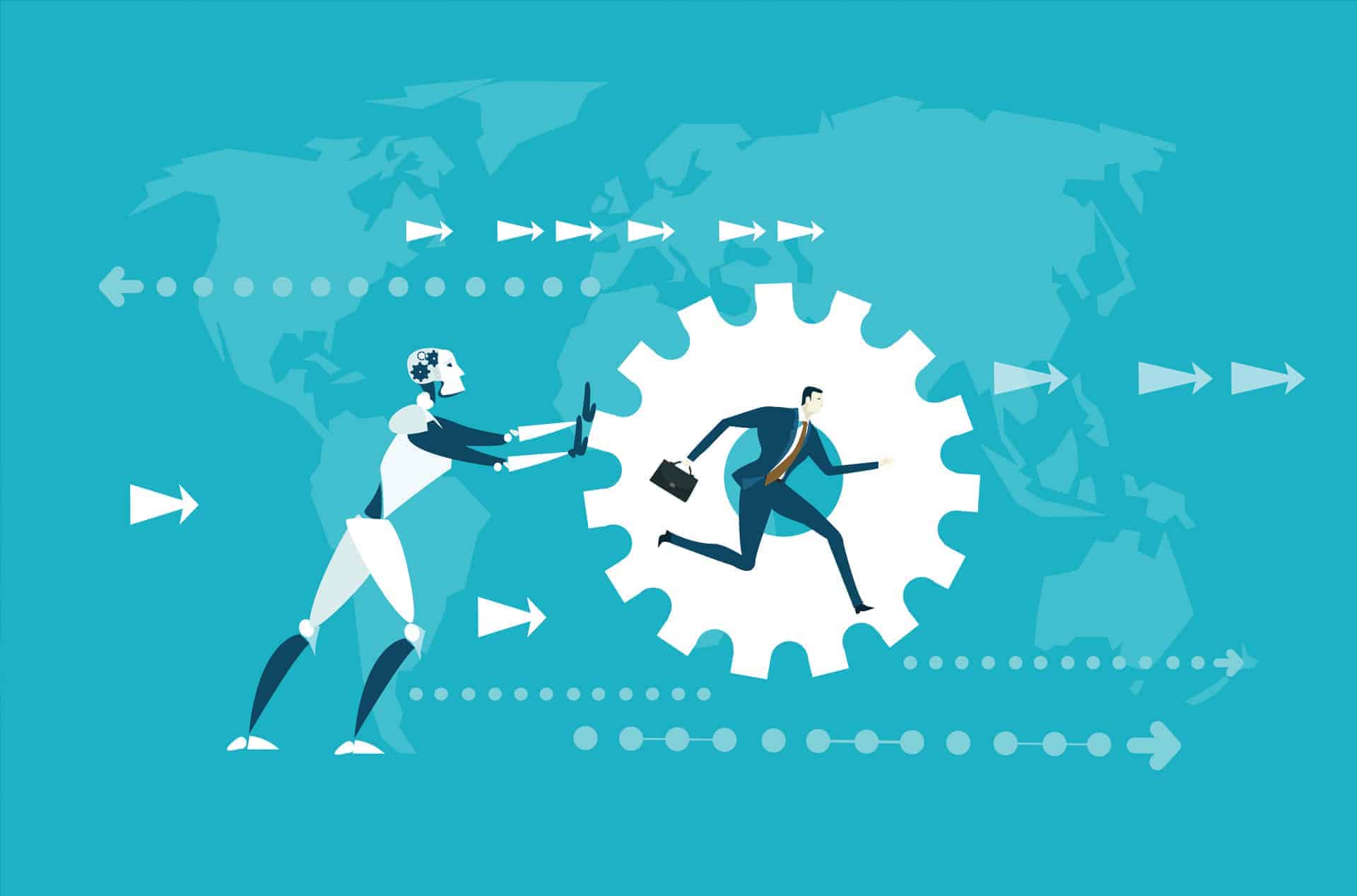
14 May Artificial Intelligence in risk Management
Is Artificial Intelligence the future of Risk Management?
In this article Risk Reward Expert and CRO Dudley Nicholls explores the expanding role of AI for risk in financial institutions and whether banks and FIs should adopt to compete
As we come out of the pandemic in 2021, and computers get ever more powerful there are a growing number of advocates for using AI models in risk management replacing some of the more traditional approaches.
AI is already being used extensively in the finance sector. Lenders are using it to calculate credit scores and banks are using it to detect fraud detection and market manipulation.
Investors have been investing heavily in recent years in computer driven hedge funds betting that advances in Artificial intelligence and big data give these funds an advantage over human traders or decision makers. A large number of hedge funds may be using AI in some form now to make predictions.
Is the next logical extension to use it far more extensively firmwide for risk modelling and is it a good thing?
What is Artificial Intelligence?
Kaplan and Haenlein define Artificial intelligence (AI) as a “system’s ability to correctly interpret external data to learn from such data and to use those learnings to achieve specific goals and tasks through flexible adaption”.
The rock -paper -scissors game shows the basic principals of an adaptive artificial intelligence technology.
Try playing Rock-Paper- Scissors against a program that uses AI and you will almost certainly lose over many games. The system learns to identify patterns in a human’s behaviour by analysing their decision strategies in order to predict future behaviour. As a human you make decisions which are not random and the computer will learn your behavioural characteristics to make better decisions. Even if you try not to follow your first instinct.
Now this is only one simple form of AI and what is AI – as opposed to what is a machine task or what could be considered simulations – is often disputed.
How can Artificial Intelligence models be used in Risk Management?
AI can be a valuable tool in any form of risk modelling that uses data, statistics and patterns. Quant traders are using AI to make investment decisions and an obvious use of AI is in Market Risk modelling.
Value at risk type models (VaR) tend to use historical data over different time periods and based of instruments historical prices and relationships make predictions with a degree of confidence of the maximum amount that can be lost. It can be argued that a flaw in these models is that it looks at where we are now but not how we got here.
Historical volatility is considered as well as historical distributions but can AI add to Market Risk modelling by adding an extra dimension of pattern recognition or signals based analysis. Take a simple example of a stock market that has risen 7 days in a row in a steady form, are you at greater risk from being short or long? Is the size of any potential move the same if the market had gone down 7 days in a row? A number of VaR models would probably say yes dependent on previous history or have a small bias in the way the market has moved but how many times do we go up or down 8 days in a row as opposed to a maximum of 7? Also has the risk increased by the market theoretically being overbought of a large down move if the next move is to be down or could it actually be less?
In looking at most VaR models, the historical moves or relationships over a number of historical days are applied to current positions. The period chosen is normally over the last one to four years (ignoring Stress Var). Expected loss looks at the scenarios outside of the VaR. What would an AI model look at?
Pure cognitive AI models will basically make predictions based off some form of pattern recognition. Based off the patterns it sees and the historical patterns it has seen an AI model would be able to predict different outcomes and like a Historical Simulation or Monte Carlo model apply probabilities. Arguably AI models which use pattern recognitions do the same thing as historical simulation type models it looks at historical scenarios but every time prices change it chooses a new set of scenarios from different points in time of what it considers the most appropriate set. It chooses the historical periods or comparable scenarios where the patterns are most closely resembled which may or may not have been recent and ignores patterns it does not think relevant. In taking into account how you got to where you are, as well as the volatility you can derive a better series of scenarios with expected probabilities. This would appear to be a step forward.
AI models are always adapting as well. Once a market opens, if historical patterns are being followed or signals are observed the probabilities will be changing all the time intra-day. Even if you have not done any new trades your risk has changed.
Those arguing against AI models at this point might say yes but, the current pattern of events is being driven by a set of circumstances different to previous times and therefore you cannot be so sure that a different pattern might not evolve. This it can be argued is especially true over the last 15 months where price changes have often been driven by Covid factors and/or more extreme economic factors. Then throw in the Reddit driven buying frenzies and Crypto currencies which are another new phenomenon and is AI really able to develop predictive properties.
AI models should adapt far quicker than traditional risk models which detect new patterns ( although using weighted data can help adapt probabilities quicker ) . AI as well can look at non-traditional market drivers. AI can also adapt for scale – historical patterns may not be as volatile but may still be valuable if scaled up.
A traditional risk model if looking at the risk in say NIO shares ( Chinese Electric vehicle company ) by looking at NIO’s history. AI models may use patterns observed in other securities , Tesla for example , to derive predictions. There have been buying frenzies in the past and new instruments , so models do have something to go on. It is an advanced piece of work to determine where cross data can and cannot be used but if say patterns were seen in growth stocks 10 years ago or the internet boom , then these may be valid in making predictions on today’s growth stocks. What you then have is a far more extensive database to draw conclusions from.
So, can AI models be extended beyond use data analysis and pattern recognition. The answer is yes. They can be easily adapted for example to incorporate economic announcements or company earnings dates. Possibly some specific events as well. Possibly Reddit posts or Elon Musk Tweets . In taking account of events it can look at periods where similar type events occurred. AI models can spot triggers and adapt predictions.
Can they be adapted to take account of changing human emotions, and should they? Again, the answer is yes (humanistic AI models are used in various fields). You need to think carefully however how to add this component such that it does not invalidate the AI analysis in the first place.
This also brings us back to what is the best use of AI models? Within a Market Risk Framework, risk managers are looking at normal risks often represented by VaR, lower probability scenarios which may be represented by expected losses and scenario analysis and extreme stress scenarios.
AI may be able to derive superior models of normal risk but is that our biggest concern? Or can AI predict extreme stresses better ?
Extreme stress events are of course very rare and somewhat unpredictable. How many times the last few years have analysts produced charts saying this pattern is just like 1987, in theory saying a 1987 crash is likely to happen only for the market to carry on. 2021 seems no different in this regard.
Can AI predict a seemingly unexpected extreme stress event more likely where humans cannot? If unpredictable it would appear unlikely – unless there are other indicators which humans are not seeing. Did hedge funds using AI perform better in the first two quarters of 2020 ? Evidence suggests they did not , actually underperforming the market in general but in the rally that followed hedge funds performed well again , outperforming.
What about the less extreme but still larger more plausible scenarios, can AI models determine the likelihood of larger moves occurring? In theory yes, providing there is precedent somewhere or signals of some kind. A number of larger moves though still are as a result of a catalyst and are often dependent on the human psyche at the time. If the human psyche can be determined by market moves prior to the event or even during then AI models can take this into account or possibly the model can be enhanced by confidence type factors.
Some large moves are predictable, if outcome A happens then the result will be X, if outcome B then Y. Look at Brexit as an example and the foreign exchange moves. Could an AI model through pattern recognition and other signal-based recognition have predicted the market reaction better following the Brexit vote or last US Presidential election? If the answer is yes then this might be a good use of AI models to predict stress related moves dependent on event outcomes.
Ultimately outside of known events should we be concerned about what the catalyst is or could we use AI models to predict based off recent moves if there were a catalyst of any kind whatever the likely magnitude of move or range of moves might be?
The same principles can be applied to operational risk trends, credit risk trends and even liquidity risk. As patterns emerge then AI models can be better equipped to spot trends, spot the unusual and provide warning signals. Spotting the unusual is arguably just data mining though.
There are clearly a range of different ways that AI can be used to make predictions and determine probabilities. Specifically, on both the financial data itself and on event probability. Ultimately it seems computers eventually beat humans as they learn. So, should we not all be investing in AI risk models?
Is Artificial Intelligence the future of Risk Management?
Computers keep getting infinitely more powerful capable of interrogating larger databases but if you have a portfolio with 50,000 or 100,000 or more different instruments / exposures then trying to determine all the relevant patterns in a short period of time is still close to impossible without using some sort of factor or principal component analysis. Then as discussed there is the overlay of event risks. One of the greatest advantages of AI is that it adjusts as patterns emerge. If you cannot run in real time then this advantage is somewhat taken away. Is the cost justifiable?
A partial high-level form of AI can be applied at a holistic level with mathematical modelling of the likely range of events. Just predicting the probable movements of the S&P 500, with a form of beta analysis and specific risk analysis is a start.
As computers speed up even more and researchers work out how the data can be applied effectively then eventually this problem will reduce. Then subject to back testing it is only a matter of time before we see risk system vendors advertising their new superior models.
In the mean-time a simplified form of AI might determine an environment. Anyone using unweighted historical data from four years right now to predict market moves is likely to be understating risk. An AI model could look at recent patterns to determine the most relevant historical period or periods of data to use. There again isn’t this what stress VaR is for?
Using AI to predict stress event probability has possibilities and no doubt as we store and cross reference more data then it may be possible to determine that either a significant market event is more probable or the size of a market move should an external catalyst occur. Then there is also the possibility of cross-referencing market data patterns into credit, operational or liquidity calculations.
Banks are already making some lending decisions based off AI analysis but it is largely still at an individual level. The next stage is undoubtably to look at portfolio risks, trends in defaults and predictions of cross defaults.
AI is becoming better at identifying fraud risks through pattern recognition and to the extent that data is available then it will be applied to a spectrum of operational risks. Just like in market risks however, how good is it at spotting extreme non-normal risks? Black Swans or White Rhinos?
Can AI models become self-defeating and increase risk?
More and more aspects of finance are being automated, often at the expense of humans. AI is a natural part of this but is it a good thing?
The World Economic Forum in August 2019 reported that the use of AI could significantly introduce troubling systematic risk. Automated High Frequency trading has led to runaway trading events such as the flash crash and as auto-investing based off AI is increasingly used then the chances of such events increase.
An observation in the trading community in 2018 was how hard it is to generate Alpha. Some Investors still trade off fundamentals and may view things over longer periods but as more traders use larger data sets and move from signal based to AI type predictive models, then everybody is trying to do the same trades. This can make predictions come true but also lead to too much one-way positioning. When a pattern is broken it can lead to a ‘non-logical’ event.
As we know financial history is littered with examples of when genius goes wrong. If risk models are based on exactly the same predictions traders are using then they are not playing the devil’s advocate role they are somewhat supposed to and significant event risk may be understated.
As AI gets used more extensively will it lead to greater or different risks? If used solely for credit analysis will it lead to discrimination against certain groups of people? As the World Economic Forum notes if large groups of people access the same data on the cloud as well a cyber security event has even greater systematic risk.
Just as machines learn about human behaviour then humans can learn about machine behaviour. Traders can learn how to drive a pattern forcing AI models to make decisions. Fraudsters if they understand how the pattern recognition works, will work out, at least for a while how to stay within a normal pattern. Then does the machine learn that things can be too normal or too unusual and ignore them.
In the immediate future there is no doubt that the use of AI will grow in the financial industry and in the long term significantly. It is hard to see why this will not include risk modelling or risk analysis. In the immediate future it’s use may grow slowly:
- Right now, it is still somewhat in its infancy and therefore it is expensive.
- 2020 reminded everybody that the world and financial markets are unpredictable. Relying on mathematical predictions of the future is fraught with danger.
- Regulators will need to accept the use of AI modelling and work out how to police it. This could be years off.
Longer term nobody wants to be seen to be behind the times. You cannot be criticised for using what is considered the best or the fastest. As financial institutions achieve success with AI then others are pressured into using it. More significantly could pressure from technology companies force banks and financial institutions into using it to ward off the threat of a Google Bank?
As AI is used more extensively within finance including risk management it needs to be used wisely. A risk manager should recognise AI limitations like any other Algorithm and be prepared to think outside of the proverbial or in this case more literal box. AI is like leverage, used the right way it can provide many benefits but used incorrectly it can multiply errors many times over.
As Jesse McWaters of the World Economic Forum notes financial companies should not be too eager to simply replace staff and that human skills will remain important even as automation becomes more widespread.
To reproduce or cite this article please contact Dudley Nicholls via email at DN@riskrewardlimited.com